Generative AI is revolutionizing pharma, transforming every stage of the drug development lifecycle with faster drug development and streamlined clinical trials. It helps analyze complex data, discover new drug candidates, and improve efficiency.
This article examines how generative AI unlocks opportunities, from repurposing existing drugs to enhancing manufacturing and patient care.
What challenges generative AI can solve in pharma
How does Gen AI benefit pharma? To get the answer to this question, let's look into issues drug development faces today.
Developing a drug takes an average of 10-15 years, with preclinical and discovery stages consuming 4-6 years. This is painfully long for people awaiting solutions to cure or at least manage their conditions. In addition, the R&D cost per drug in the United States is approximately $2.3 billion (source: Deloitte), exacerbated by trial-and-error lab experiments. To add insult to injury, only 1 in 10 drugs make it to market, often after extensive setbacks.
Generative AI in pharma can solve this issue, as it reduces time spent on experimentation. AI can also identify and rank drug candidates faster by analyzing large datasets of existing compounds. Overall, Generative AI in pharma can reduce drug discovery timelines by up to 70%, same for drug development costs (Source ).
AI also tackles clinical trial issues. With traditional methods, finding eligible participants for clinical trials can take years. Meeting strict criteria and finding enough volunteers takes a lot of time and effort. The trials are also costly (40–60% of total drug development costs). Poor patient engagement leads to trial failures and wasted resources.
AI speeds up the process of patient recruitment by analyzing medical records to identify eligible participants up to 5x faster. AI tools powered by predictive analytics in pharma can also reduce trial start-up times. Molecules identified through AI exhibit greater success in early clinical trials, with Phase 1 trials showing success rates between 80-90%, significantly higher than the historical industry averages of 40-65% (Source ).
Some of the other challenges that generative AI for pharma is prepared to solve are:
- Personalization of medicine
- Fluctuations of supply chain management
- Enhancement of marketing and sales campaigns
- Big data analytics on pharma
Gain a competitive edge with our comprehensive AI guide
Access adoption strategies, risk mitigation tips, and future trends
Get the knowledge you need to lead with Generative AI
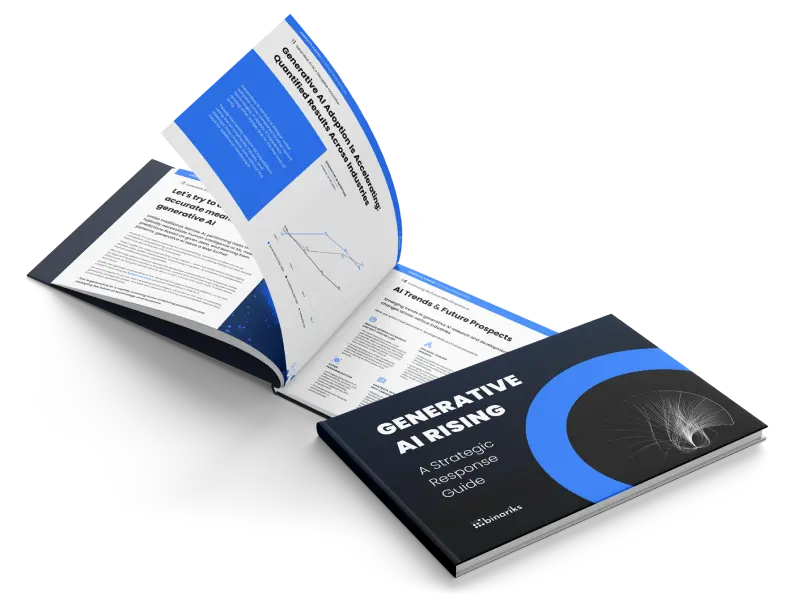
Practical applications of GenAI in pharma
Now that we have established what issues generative AI in pharma will help solve, let's look at how different gen AI use cases in the pharmaceutical industry work.
Predicting drug-target interactions
Generative AI works well for predicting drug-target interactions and discovering drug side effects.
- Mechanism: Using deep learning models, AI predicts how a drug molecule interacts with specific biological targets, such as proteins or receptors. These interactions help identify potential drug candidates early in the AI in pharma R&D process.
- Impact: This accelerates preclinical studies, reduces trial and error in the lab, and minimizes R&D costs.
- Example: BenevolentAI uses generative models to uncover novel drug-target interactions, leading to breakthroughs in treating diseases like ALS and inflammatory disorders. In partnership with the Sheffield Institute for Translational Neuroscience (SITraN) at the University of Sheffield, BEN-34712 showed promising results in early testing for ALS (amyotrophic lateral sclerosis) (Source ). It protected nerve cells in lab-grown models derived from ALS patients, working effectively for both common and inherited forms of the disease. In addition, when tested on a mouse model of ALS, the treatment demonstrated strong engagement with its target in the brain and provided protective benefits after 50 days of treatment.
Drug optimization
Generative AI refines molecular designs to create more effective and well-tolerated drug candidates. It can also foresee the potential issues with the quality of the drug and improve drug audits.
- Mechanism: AI algorithms analyze molecular properties and suggest structural modifications to optimize drug performance. This involves refining molecular properties to enhance effectiveness and suitability for therapeutic use.
- Impact: AI-driven optimization reduces the time spent on chemical synthesis and testing, cutting months from the R&D timeline. In fact, AI-driven optimization can reduce this time by 50% or more.
- Examples:
Drug repurposing
Generative AI in pharma identifies new therapeutic uses for existing drugs by studying drug compound databases. It can also act the other way around, starting with a disease and looking for existing drugs that can be repurposed. Side-effect identification also matters in this process. This helps to bypass many hurdles of traditional drug development.
During drug repurposing, pharma-generative AI can even suggest what changes need to be made to use existing drugs for new biological targets.
- Mechanism: AI scans vast datasets of known drugs and diseases, looking for matches between drug properties and new indications.
- Impact: This significantly reduces time-to-market since repurposed drugs often have established safety profiles.
- Example: Generative AI was instrumental in identifying the potential of Remdesivir for treating COVID-19, accelerating its deployment during the pandemic (Source ). It did so by analyzing its interaction with the virus.
Automation of documentation
Regulatory documentation and clinical trial reporting are time-consuming yet critical processes in pharma. Software development for pharma can automate these tasks with generative AI.
- Mechanism: AI generates and refines essential documentation based on input data.
- Impact: Automation improves accuracy and efficiency while keeping processes aligned with regulations.
- Example: Pfizer uses AI-powered tools to automate clinical trial documentation, saving thousands of hours annually (Source ). AI is also used to translate documentation into different languages.
De novo drug design
Generative AI enables the creation of entirely new molecules from scratch.
- Mechanism: AI uses advanced algorithms, such as Generative Adversarial Networks (GANs), to design molecules that meet specific criteria. These models are trained on vast datasets of molecular information to generate structures with desired properties.
- Impact: This approach opens up new possibilities for drug discovery, particularly in addressing rare or treatment-resistant diseases, by identifying candidates that traditional methods might miss.
- Current progress: While no AI-designed drugs have yet completed all clinical trial phases or reached market approval, several are actively undergoing trials. These include the examples mentioned earlier, demonstrating the growing potential of AI in drug development.
Enhancing patient recruitment for clinical trials
AI streamlines the recruitment process by identifying suitable candidates for clinical trials. Generative AI for pharma can also simulate different scenarios, like reactions to different doses and overall response to treatment. Gen AI in pharma also simulates the design of trials.
- Mechanism: Generative AI pinpoints individuals who meet the trial criteria by analyzing medical records and genetic data.
- Impact: This reduces recruitment time and ensures trial diversity, leading to more reliable results.
- Example: AI-driven recruitment tools have reduced trial start-up times by up to 30%, as reported by several CROs (Contract Research Organizations) (Source ).
Optimizing pharmaceutical manufacturing
Generative AI enhances manufacturing processes by predicting equipment failures and optimizing production parameters.
- Mechanism: AI models simulate production environments to optimize factors like temperature, pressure, and mixing times for drug synthesis.
- Impact: This prevents costly downtime by improving efficiency and maintaining consistent product quality while minimizing waste.
- Example: Novartis uses AI to optimize vaccine production, reducing manufacturing cycle time by 20%. It can potentially reduce drug manufacturing cycle time by as much as 90% (Source ).
Virtual drug testing
AI conducts in-silico experiments to predict a drug's efficacy and safety before physical testing.
- Mechanism: Generative models simulate biological environments, allowing virtual testing of molecular interactions and side effects.
- Impact: This reduces dependency on animal testing and lowers the risk of failure in later trial stages.
- Example: AI-driven virtual testing allowed Moderna to optimize its mRNA vaccine candidates, significantly speeding up the COVID-19 vaccine development and distribution (Source ).
Drive pharma innovation with Binariks' tech expertise!
Real-life examples of generative AI solutions for pharma
Though we have already tackled pharmaceutical generative AI applications, it is worth having a detailed look into how companies use generative AI for pharma, including an example of our use case.
Insilico Medicine
Insilico Medicine developed Chemistry42, an AI-powered platform that predicts drug-target interactions to accelerate drug discovery. The platform combines pharma generative AI with physics-based methods to design and optimize novel compounds. In total, it uses over 30 AI models (GANs, autoencoders, etc.) to create molecules with specific properties.
Their generative AI model designed INS018_055, a drug candidate for idiopathic pulmonary fibrosis, progressing to Phase I trials in just 30 months—half the traditional timeline. As of early 2025, the drug is currently undergoing Phase II clinical trials in the United States and China.
The discovery reduced costs by 90%, spending approximately $40 million instead of $400 million (Source ).
Moderna
The company used AI to optimize mRNA sequences for their COVID-19 vaccine. The sequence used for the vaccine was completed in less than 2 days after the genetic sequence of SARS-CoV-2 was published in January 2020, thanks to gen AI in pharma (S ource ). It also assisted with scaling up manufacturing to meet the global demand.
All in all, the total duration of trials took just 9 months, and the vaccine hit the market in December 2020, which helped mitigate the disrupting global health crisis that was COVID-19.
Healx
Healx uses its AI platform, Healnet, to find new uses for existing drugs to treat Fragile X syndrome (FXS), a leading inherited cause of autism and learning disabilities.
Among the drugs identified was HLX-0201, originally a nonsteroidal anti-inflammatory. Preclinical studies showed it could influence behaviors linked to FXS. In October 2021, Healx received FDA approval to begin Phase 2a clinical trials for HLX-0201 in FXS patients (Source ).
Issues of GenAI in the pharmaceutical business
For all its potential, gen AI solutions for pharma companies come with challenges, particularly as many companies overestimate their vast capabilities and falsely attribute abilities it does not yet have.
- The overall quality of pharma training datasets
Generative AI models are always trained on large datasets. However, training data is scarce in the healthcare industry for several reasons. It is limited by privacy, the high cost of data collection, and limited data for rare diseases.
Moreover, pharma data is often unstructured or spread across multiple formats (e.g., research papers, lab notes, EHRs), making it challenging to aggregate and use for AI training. Only 1/4th of pharmaceutical data is estimated to be available for research (Source ).
- Potential bias
The performance of an AI model can reflect the biases already present in a database. At times, AI models can introduce their own biases if it is made possible by their algorithmic design. Some of the few potential existing biases that generative AI use cases in pharma can reflect are:
- Women are often underrepresented in clinical trials for cardiovascular drugs despite it being one of the main causes of female death.
- Black patients are underrepresented in cancer and hypertension trials. Notably, there have been findings that they are underrepresented in trials by the National Cancer Institute (NCI) (Source ).
- Asian populations often metabolize certain drugs differently due to genetic variations. For example, they require a lower dosage of a blood thinner Warfarin.
- Older adults are underrepresented in trials despite being the primary users of many drugs.
The good news is that the personalization that comes with generative AI in pharma has the potential to solve these issues with clinical trials.
- Overreliance on Gen AI in pharma without considering limitations
While Gen AI in pharma is a disruptive force, it cannot solve everything, and overreliance on it will indeed cause an oversight of nuanced factors. First, Gen AI solutions for pharma companies cannot deliver value alone without an investment in proper data architecture. Building a Gen AI model is not everything – most of the work is adapting the company's knowledge base and use cases to this data.
Pharma data includes information on complex biological systems, which means that the model must be very comprehensive to actually understand the entire system and the sum of its components.
Finally, even the most comprehensive Gen AI model can end up experiencing hallucinations, so checking everything is essential, especially in the high-stakes healthcare industry.
What is the future of GenAI in the pharmaceutical industry?
Generative AI for business is a perfect investment for any pharmaceutical company with resources for such investment.
The global AI in healthcare market was valued at $8.23 billion in 2020 and is projected to reach $194.4 billion by 2030, growing at a CAGR of 38.1%, an enormous number that few industries can come near in 2025 (Source ).
Part of why the market is growing at such an unprecedented pace is the increase in pharmaceutical R&D spending, which totaled approximately $238 billion in 2022 (Source ). There is also vast potential from the shift towards precision medicine, which also helps move generative AI forward. For those worried about uncertain AI regulations, it is worth knowing that it will soon not be the problem anymore, as frameworks for approval of AI-driven innovations are now being created.
Pharma-generative AI is an all-around great solution as AI reduces the average cost of drug development, shortens the drug discovery process from years to months, and allows for solutions that can be scaled across various markets.
Early adopters of AI will gain a competitive edge, and there is still time to do this even though AI is gaining more prominence.
In the near future, generative AI is expected to become integral from early-stage drug discovery to post-market surveillance, and it will gain more prominence in clinical trials. Drug repurposing will be one of the market's key segments, given how effective AI is at drug repurposing.
As a pharmaceutical business, you need the right tech partner to navigate the complexities of implementing generative AI effectively, ensuring the technology aligns with your goals and delivers measurable outcomes.
Binariks has firsthand experience building AI solutions for healthcare and life sciences industries, streamlining patient recruitment, and improving data analysis processes. We help companies cut trial start-up times and make faster, data-driven decisions with our AI development services backed by years of working on healthcare technology innovations.
Contact us to unlock the full potential of generative AI and drive innovation in your pharmaceutical operations.
Share