Artificial intelligence has revolutionized the healthcare sector in many ways, with AI in medical imaging being one of its most groundbreaking applications.
AI serves as a second reader for radiologists, providing a reliable cross-check for detecting anomalies in medical images. It can also handle massive amounts of data and extract hidden information from the scans that are invisible to the human eye.
Despite the revolutionizing nature of medical imaging with AI, data security, quality, and processing issues persist. In this article, we uncover everything about AI and medical imaging.
How did it work before?
Before medical imaging AI integration, medical imaging and radiology relied mainly on manual processes and the expertise of radiologists to interpret images. Imaging devices such as X-rays, CT scanners, and MRIs require technicians to make manual adjustments to obtain clear images.
Acquiring high-quality images took considerable time. Radiologists manually examined images to identify abnormalities. Measurements and quantifications, such as tumor size or organ volume, were performed manually, which could be time-consuming and prone to inaccuracies.
Diagnoses were mostly based on the radiologist's experience and judgment. Prioritizing urgent cases over routine ones was less systematic, sometimes leading to delays in diagnosing critical conditions. Detecting tumors that were yet to develop was, in most cases, out of reach as human expertise could not tackle the subtle signs needed to predict these conditions.
Before the invention of AI, medical imaging issues included human errors, quality inconsistency between radiologists depending on their skill and expertise, fatigue from high workloads, time-consuming analysis processes, and a lack of advanced analytics tools. All of these issues are still present today, at least to some extent, but AI-enhanced medical imaging is slowly on track to solve them.
The growth of AI in medical imaging market
First and foremost, the growth of AI for medical imaging is stimulated by the growth of AI in the healthcare market in general. The global healthcare market is currently estimated at USD 20.9 billion in 2024, and is expected to reach USD 148.4 billion by 2029. AI-based medical imaging, along with drug development and precision medicine, is a huge part of that market.
Here are some stats on AI in the medical imaging market:
- The global AI in the medical imaging market was valued at approximately USD 1.01 billion in 2023. It is projected to grow at a compound annual growth rate (CAGR) of 34.8%, reaching about USD 11.76 billion by 2030 (Source ).
- In the U.S., the AI in medical imaging market was valued at USD 395 million in 2023 and is expected to grow at a CAGR of 33.2%, reaching around USD 3.14 billion by 2030.
- The market growth is primarily driven by the increasing adoption of AI technologies to manage large and complex datasets, improve diagnostic accuracy, and enhance the efficiency of radiology workflows. AI's ability to automate and optimize various aspects of medical imaging processes also contributes to its rapid adoption.
- According to a study published in Healthcare (Basel) magazine, the rate of CT scans has doubled since 2010. There are various reasons, including an aging population, challenges like the COVID-19 pandemic, and the growing availability of these services. AI is helping to improve imaging technologies to unprecedented levels. As of 2023, the FDA has approved 691 medical devices, many of which are in radiology (Source ).
Applications of AI in medical imaging
Here is a brief overview of AI applications in medical imaging to treat various conditions.
Cardiovascular conditions
There are plenty of cardiovascular applications for AI and medical imaging:
- AI-powered medical imaging analysis can better visualize a heart with the coloration of heart chambers on grayscale echocardiology images.
- AI algorithms can analyze chest X-rays to detect left atrial enlargement, a precursor to various cardiac issues. For example, researchers at UCSF developed an AI model that accurately identifies this condition (Source ).
- In another example, Philips' IntelliSpace Portal uses AI to automate the analysis of the aortic valve, which helps diagnose conditions like aortic stenosis (Source ). Philips also develops models for heart coloration and heart 3D visualization.
Neurological conditions
In neurological conditions, AI in existing radiology is increasingly used for diagnosing Alzheimer's and ALS:
- Mount Sinai Health System uses AI models to analyze brain scans and identify early signs of Alzheimer’s disease. This technology provides insights into disease progression (Source ). Different algorithms developed for the early detection of Alzheimer's disease can help identify it by very subtle signs, like the decrease in glucose uptake. These studies are very important as they can aid physicians in stopping the progression of this incurable disease.
- Likewise, ALS is an untreatable condition, and early diagnosis is critical for a long-term care plan. IBM Watson Health uses AI to analyze MRI images to diagnose ALS. It helps deal with false positives, which are very common for this diagnosis.
Cancer screening
- AI enhances the accuracy of mammograms by identifying microcalcifications that indicate breast cancer. For instance, a study by the Radiological Society of North America demonstrated how AI reduces false positives and ensures timely treatment (Source ).
- Medtronic's GI Genius uses AI to assist in colonoscopies by identifying polyps in real time (Source ). This is the first device available on the market without any analogs.
Brain tumors
- AI models can accurately classify brain tumors from MRI images. A study showed that AI achieved a 98.56% accuracy rate in classifying brain tumors (Source ). They can also classify brain tumors faster than traditional models, just in minutes. AI can also tackle false-negative results and images where the signs of cancer are minimal and cannot yet be detected by conventional means.
- A UK study developed an AI model to non-invasively classify brain tumors in children, providing a safer alternative to biopsies, which can be especially challenging for kids (Source ).
Fractures and musculoskeletal injuries
- Zebra Medical Vision's AI tool analyzes X-rays to accurately detect hip fractures, ensuring timely treatment and better outcomes for elderly patients.
- A Stanford University study utilized AI to detect subtle fractures and musculoskeletal injuries from X-rays and MRIs, improving detection rates and patient care (Source ).
- Many vertebral fractures go unnoticed on traditional CT scans (Source ). Deep learning algorithms can solve this issue.
6 Advantages of AI for medical imaging
Let's look into how AI improves medical imaging across all its applications. Here are the benefits of AI in medical imaging:
1. Improved diagnostic accuracy
- AI algorithms can analyze medical images with high precision, reducing the chances of human error and oversight. Studies show that AI can help detect conditions that human radiologists might miss.
- AI can identify early signs of diseases, such as cancer, in medical images, enabling earlier intervention and better patient outcomes. Some of the findings AI-driven medical imaging diagnostics can provide are not yet visible to the human eye. AI algorithms can be specifically trained to identify particular signs of disease.
- For example, Google Health developed an AI model that analyzed mammograms and could predict breast cancer development up to five years in advance. The AI model identified subtle tissue changes in mammograms that are often invisible to the human eye. The study showed that AI could reduce false positives by 5.7% and false negatives by 9.4% compared to radiologists (Source ).
- AI for medical imaging assists in precision medicine. For example, it can differentiate between different types of lung cancer. Besides, it can more accurately predict the outcome of the disease and the survival rate based on grade and stage.
- AI can perform opportunistic imaging, analyzing images for additional health clues beyond the initial diagnostic purpose. This can lead to early detection of conditions not suspected initially. For example, AI can identify patients at risk of heart attacks and strokes by analyzing chest X-rays for signs beyond lung health.
2. Increased efficiency
- AI speeds up the image analysis process, allowing radiologists to review more cases in less time and reveal diagnoses faster. This efficiency is particularly beneficial in high-volume settings. Data science can be used to analyze large datasets of patient information.
- For example, researchers at Facebook AI Research (FAIR) and NYU School of Medicine developed fastMRI, an AI model that accelerates MRI scans. The AI reduces MRI scan times from 60 minutes to 15 minutes without compromising image quality (Source ).
- AI can generate preliminary reports, which radiologists can then review and finalize, reducing the time required for documentation.
3. Enhanced workflow
- AI systems can prioritize urgent cases, ensuring that critical conditions are addressed promptly. This triage helps manage workload and improves patient care.
- AI seamlessly integrates imaging data with electronic health records, providing a comprehensive view of patient history and aiding in more informed clinical decision-making.
4. Cost-effectiveness
- By automating routine tasks, AI allows radiologists to focus on more complex cases, optimizing the use of human resources. This can lead to cost savings for healthcare facilities.
- Improved image quality and accuracy mean fewer repeat scans, reducing costs and patient radiation exposure.
5. Consistent quality
- AI provides consistent analysis and interpretation of medical images, reducing variability between radiologists and improving overall diagnostic quality.
- AI can be a valuable tool for training radiologists, providing feedback, and highlighting areas for improvement.
6. Advanced capabilities
- AI excels in analyzing complex images and detecting subtle patterns that might be challenging for human radiologists. This capability is especially useful in fields like neurology and oncology. While standard medical imaging cannot determine the percentage of living cancer cells, AI in medical imaging can.
- AI-based medical imaging is used in predictive analytics to determine disease progression and patient outcomes based on imaging data and other clinical factors, aiding in treatment planning and follow-up care.
Will adopting GenAI mark the next chapter for your business?
Download our free whitepaper now to find out.
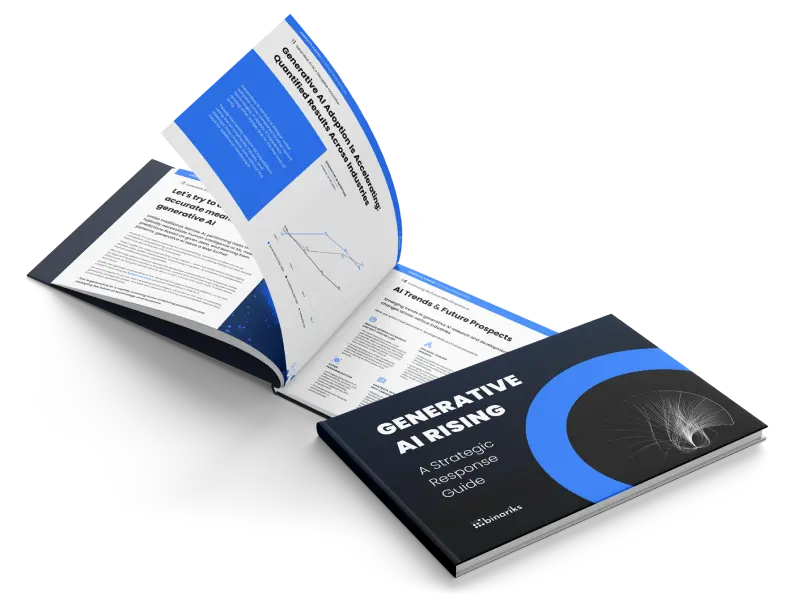
What other technologies help AI?
You can get the most out of artificial intelligence in biomedical imaging by combining it with other technologies. Let's dive into how these collaborations enhance radiology:
Big data
Collaboration between AI in medical imaging and big data is especially fruitful compared to all other technologies. Big data science can do a lot for medical imaging.
AI algorithms can identify abnormalities, not immediately, if at all, visible to radiologists. The systems can be trained to recognize the signs of specific conditions. Moreover, AI in radiology workflows with data science is trained to analyze vast information and cater to patients' personalized treatment plans.
Data science is also a tool in predictive modeling for identifying potential health outcomes based on a combination of comprehensive information (medical imaging, lab tests, and genetic information).
3D and VR
3D and VR in healthcare are mainly used for 3D medical imaging with AI. AI can process 2D medical images to create detailed 3D reconstructions of MRIs and CT scans.
Regular 2D MRIs lack spatial dimensions, so without AI, doctors have to use their expertise to comment on the elements of the image they cannot actually see. Once the 3D model of the scan is created, the doctors can even use a VR headset to examine all the details of a 3D model. It does not even have to be a specialized VR model; a regular commercial headset like VisionPro serves this purpose.
The visualization from AI-powered medical imaging analysis with a VR headset is very helpful when preparing for surgery, especially in complex cases like brain tumor resections.
Wearables
While data from wearables can be integrated with imaging data to provide a comprehensive health profile and personalized treatments, the solutions wearables can now bring for AI in medical imaging go beyond just monitoring basic health metrics like heart rate or insulin levels.
More specific AI medical imaging solutions are now entering the market, like the device for brain imaging from Washington University in St. Louis (Source ). The device uses LED lights to observe the patient's regular brain activity and activity during abnormal events like seizures.
Simultaneously, other wearable scanners are being developed for the detection of bladder and kidney diseases and breast cancer.
Augmented intelligence
Augmented intelligence combines human expertise with AI to create a decision-support process that improves clinical decision-making accuracy and speed. These systems provide recommendations based on AI analysis while allowing clinicians to apply their judgment and expertise. As a result, the diagnosis is a collaborative effort between the doctor and the AI system.
For example, a specific augmented intelligence solution for radiology Aidoc uses AI to analyze medical images and detect abnormalities. It helps radiologists by prioritizing cases that need immediate attention based on the severity of findings (Source ).
The program scans CT images for signs of intracranial hemorrhage, pulmonary embolism, and cervical spine fractures. Another popular augmented solution is Arterys, a program that analyzes cardiac MRI images to quantify blood flow and assess cardiac function.
Problems of AI integration in medical imaging and strategies to overcome them
Regardless of all the exciting opportunities that AI in clinical imaging brings to the table, there are challenges with data processing, security, and quality of the outputs. Here are some of the most common challenges and ways to overcome them:
1. System compatibility
- Many healthcare facilities use legacy systems that are not designed to integrate easily with modern AI technologies. Ensuring seamless interoperability between AI tools and existing Picture Archiving and Communication Systems (PACS), Radiology Information Systems (RIS), and Electronic Health Records (EHRs) can be complex and costly.
- There is also a lack of standardization in AI tools, which can lead to difficulties in integrating these technologies with different systems and workflows used by various healthcare providers.
Solution: Implementing middleware software can bridge the gap between legacy systems and new AI tools, facilitating smoother integration without overhauling existing systems while industry-wide interoperability standards are still developing.
2. Data processing
- AI algorithms require large, high-quality datasets for training. However, medical imaging data can be inconsistent, incomplete, or of varying quality, posing a significant challenge for practical AI training.
- Properly labeled data is crucial for training AI models. Manual labeling is time-consuming and resource-intensive, and there can be inconsistencies in how different radiologists label data.
Solution: As the healthcare industry transforms, more datasets will soon adhere to high-quality standards. However, utilizing AI and machine learning for automated data labeling can reduce the time and resources required even now.
3. Aligning medical guidelines with AI outputs
- Many AI-based medical imaging applications provide estimates of how likely a certain patient is to develop a condition. For example, let's say that a specific endometrial growth is 12% likely to be malignant. While a human radiologist can recommend a biopsy based on this information, an AI system may find the risk of developing a condition too insignificant.
Solution: The solution to this issue is the continuous collaboration between human professionals and AI in existing radiology. Artificial intelligence is a second reader and not a replacement for a doctor. The practice of actual doctors should be used to train AI in radiology workflows.
4. Reliability and security
- AI models can inherit biases in the training data, leading to inaccurate or biased results. This is especially concerning in diverse populations where biased algorithms may not perform well across different demographic groups. Studies reveal that AI models can use race, gender, and other inherent characteristics as shortcuts to diagnoses that might not be accurate (Source ).
- Ensuring AI tools meet regulatory standards and obtaining necessary approvals according to the regulation of AI in healthcare (e.g., FDA approval in the U.S.) can be a lengthy and challenging process.
- Medical imaging data is sensitive and must be protected against cyber threats.
Solution: Implementing strategies to detect and mitigate biases in AI models, such as diverse training datasets and continuous performance monitoring across different demographic groups, can help. It is also important to implement robust cybersecurity protocols, such as encryption, access controls, and regular security audits, to protect sensitive data.
5. Implementation and maintenance
- The initial investment for integrating AI technologies into medical imaging workflows can be high, including software, hardware, training, and ongoing maintenance costs.
- Healthcare professionals need adequate training to use AI tools effectively. Resistance to adoption can result from unfamiliarity with the technology and concerns about its impact on workflows and job roles.
- As new data and imaging techniques emerge, AI models need continuous updates and retraining to remain accurate and effective. This requires ongoing resources and commitment from healthcare organizations.
Solution: Conducting detailed cost-benefit analyses to justify initial investments is a good way to start, just as developing comprehensive training programs for healthcare professionals. You should also have a plan for continuous maintenance and updates for your AI systems.
6. Ethical and legal concerns
- Determining accountability for AI-driven decisions can be complex. Questions arise about who is responsible if an AI system makes an incorrect diagnosis or recommendation.
- The ethical considerations of utilizing AI in healthcare, including obtaining patient consent and upholding transparency in AI decision-making processes, must be carefully evaluated.
Solution: More precise ethical and legal standards are the way to go here, and only developing these standards will help solve these issues.
Take your software to new heights with AI/ML solutions
Our experience
Integrating AI medical imaging solutions into your healthcare organization's workflow requires an IT team's dedicated effort. At Binariks, we have the appropriate experience to address data-related challenges in AI-driven medical imaging diagnostics. With over eight years in healthcare software development services , here is what we can do for your healthcare organization:
- Offer options for cloud storage of data
- Assist in managing large datasets required for training IT models
- Offer middleware solutions for integration with EHRs and other existing IT systems
- Develop customized solutions for specific clinical needs
Final thoughts
AI is making significant strides in medical imaging. Acting as a second set of eyes, AI helps radiologists catch anomalies that might be missed and manage large datasets efficiently. This advancement allows for early disease detection, better treatment planning, and improved patient outcomes.
However, integrating AI into healthcare systems has challenges like compatibility, data quality, and security concerns. There are also issues with AI biases and ethical implications that need addressing. Turning to a qualified IT team for solutions to these issues can help take advantage of the benefits of AI in healthcare for imaging.
The future of AI in medical imaging is promising. AI could enhance personalized medicine, tailoring treatments based on a patient’s genetic profile and lifestyle. Predictive analytics might forecast disease progression. AI combined with wearable tech could enable continuous health monitoring, offering real-time diagnostics and early warnings.
While there is still a way to go for this technology to become perfect, it will surely change healthcare as we know it.
Share